Enhancing Airflow for Analytics, Data Engineering, and ML at Wikimedia
- Track: Data Analytics
- Room: UB5.132
- Day: Saturday
- Start: 17:25
- End: 17:55
- Video only: ub5132
- Chat: Join the conversation!
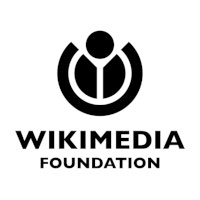
The Wikimedia Foundation supports hundreds of thousands of people around the world in creating the largest free knowledge projects in history. In order to do this we run on-premise infrastructure at significant scale, using almost exclusively free and open-source components. Our data processing and real-time analytics requirements are constantly evolving and our Data Platform Engineering teams face complex challenges in the fields of data-engineering and machine-learning, as well as the operational workload of supporting these systems in production.
Wikimedia’s data platform today runs on some of the most vital open source projects such as Hadoop, Kubernetes, Ceph, Druid, Cassandra, Spark, Hive, Iceberg, Flink, Presto, Jupyter, MariaDB, PostgreSQL, Superset, and Airflow.
We started working with Airflow in 2019, when it was still an Apache incubator project. Over the past five years our deployments have matured and we succeeded in migrating the last of our Oozie-based workflows to Airflow in mid-2023. In addition, we offer Airflow services to other data-focused WMF engineering teams, in order to facilitate a self-service approach to data pipelines.
During 2024, the Data Platform SRE team undertook a major project to enhance our Airflow services by migrating them from bare-metal and VMs to our on-premise Kubernetes and Ceph clusters. This burgeoning integration between Airflow, Kubernetes, Spark, and Ceph has enabled us to broaden the scope and applicability of Airflow to include ML model training and data publishing workloads, plus more in future.
This is an account of how we got here, the challenges we overcame and where we plan to go from here.
Speakers
![]() |
Ben Tullis |
![]() |
Balthazar Rouberol |