Bringing Machine Learning Renewable Energy Forecasting Models to the Open Source Community - data engineering and other challenges implementing large ML models
- Track: Energy: Accelerating the Transition through Open Source
- Room: H.2214
- Day: Sunday
- Start: 14:35
- End: 14:50
- Video only: h2214
- Chat: Join the conversation!
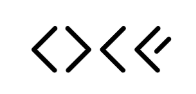
Solar energy is predicted to be the largest form of power generation globally by 2040 and having accurate forecasts is critical to balancing the grid. Unlike fossil fuels, renewable energy resources are unpredictable in terms of power generation from one hour to the next. In order to balance the grid, operators need a close estimate of when and how much solar and wind power will be generated on a given day.
Open Climate Fix (an open source AI company) developed and deployed PVNet, a large ML model which forecasts solar generation for the next 36 hours. The forecasts are used by the UK electricity grid operator for real-time decision making and for reserve planning. These forecasts can save 200,000 tonnes of CO₂ and £20 million per year.
But how do we make sure the model is usable by the Open Source community? We will explore the challenges of accessing 100s of TB of Numerical Weather Prediction (NWP) datasets, and the challenges of data engineering these large NWP datasets. We will also talk about the challenge of working with these large ML models and what we are doing to enable the Open Source community to benefit.
Open Climate Fix is an open-source not for profit company using machine learning (ML) to respond to the need for accurate renewable energy forecasts. Connecting energy industry practitioners with ML researchers doing cutting-edge energy modelling is our aim, and one way we seek to do this is by making much of our code open-source.
Speakers
Peter Dudfield |