Explainable forecasting from big weather data: rapid and sustainable solutions
- Track: HPC, Big Data & Data Science
- Room: UB5.132
- Day: Sunday
- Start: 10:00
- End: 10:25
- Video only: ub5132
- Chat: Join the conversation!
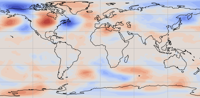
We present DynaModERA, an open-source Python package to perform Dynamic Mode Decomposition (DMD) at scale on the publicly available ERA5 dataset, the fifth generation ECMWF atmospheric reanalysis of the global climate covering the period from January 1940 to present. DMD is a popular technique for data-driven modeling of a variety of dynamical systems due to its simplicity and interpretability. In contrast to state-of-the-art deep learning models for data-driven weather prediction, such as those developed by NVIDIA, Huawei and Google DeepMind, DMD is a computationally inexpensive algorithm that provides a best-fit, linear characterization of a non-linear dynamical system, and generates explainable and interpretable results in the form of spatial modes with temporal evolution. These modes often have physical meaning that align with the underlying physics of the problem.
A common limitation of DMD is its inability to handle large datasets. DynaModERA addresses this challenge by enabling DMD on big weather data through the generation of low-rank approximations of ERA5 and the construction of smaller DMD models for different temporal subsamples. These models are then combined into a unified framework, allowing for stable predictions across all time scales. DynaModERA also provides a comprehensive pipeline for the entire process, including downloading appropriate ERA5 slices from the cloud, data versioning and tracking using Data Version Control (https://dvc.org/) , producing low-rank approximations at scale, and generating DMD models and predictions. By applying DMD to extensive portions of the ERA5 dataset, DynaModERA not only establishes a benchmark for comparing more advanced data-driven weather prediction models but also provides valuable physical insights through DMD modes, which can be used as input features for deep learning models.
Speakers
![]() |
David Salvador-Jasin |